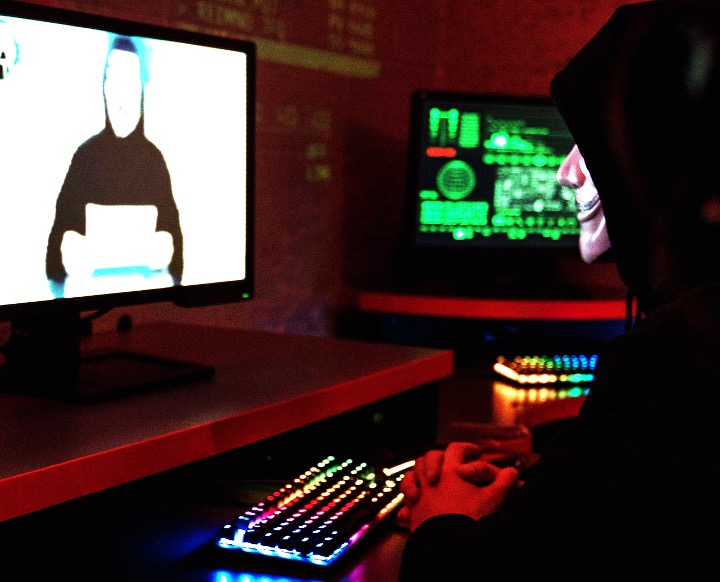
Cybersecurity has been compared to an arms race – as monitoring and malware detection evolve, so do the tactics of cyber adversaries. One of the most concerning developments in recent years is the emergence of adversarial machine learning (AML) which accelerates malware development. This phenomenon has profound implications for IT budget holders, as it necessitates a reevaluation of cybersecurity strategies and resource allocation to effectively counter the rise of malicious software development.
What is Adversarial Machine Learning?
Adversarial machine learning is the manipulation of machine learning models by adversaries to evade detection or cause misclassification. This is achieved by exploiting vulnerabilities in the underlying algorithms, data, or training processes to generate adversarial examples. Adversarial examples can be used as inputs that are carefully crafted to deceive or subvert a model.
EXAMPLE:
Adversarial machine learning techniques can be categorized into various methods. These include gradient-based attacks, where adversaries perturb input data to maximize the model’s prediction error, and model inversion attacks, where adversaries attempt to infer sensitive information about the training data. An early, and well-publicized example was the Kyushu University researchers who in 2017 managed to ‘fool’ many image analysis algorithms. Single-pixel changes in images resulted in their misclassification – a turtle was recognized as a rifle and a stealth bomber was identified as a dog.
Adversarial Machine Learning and Malicious Software Development
The integration of adversarial machine learning techniques into the realm of cybersecurity has empowered cybercriminals to develop more sophisticated and evasive forms of malware. By leveraging adversarial examples, adversaries can evade detection by traditional security measures and exploit vulnerabilities in machine learning-based defenses.
Additionally, chatbots such as WormGPT, FraudGPT, DarkBert, or DarkBART provide ways to subvert the security measures of public models for a small monthly subscription (between 60€ and 200€ per month).
EXAMPLE:
Adversarial machine learning has been used to create stealthy malware variants that can bypass antivirus software, intrusion detection systems, and other security controls. They do this by exploiting weaknesses in machine learning models used for threat detection. Deep statistical analysis of malware variants showed early promise as a method of detecting adversarial malware inputs, but this approach is now viewed only as a partial solution.
Implications for IT Budget Holders
The proliferation of adversarial machine learning poses significant challenges for IT budget holders, as it introduces new complexities and uncertainties into the cybersecurity landscape. Addressing this threat requires strategic investments in advanced security technologies, threat intelligence, and workforce training to enhance detection and response capabilities.
EXAMPLE:
According to a 2020 survey conducted by Accenture, 83% of cybersecurity professionals believe that adversarial machine learning will have a significant impact on their organization’s cybersecurity strategy in the next five years. More recently, Accenture predicts over 15% increase in application- and data-security spending through 2025.
Addressing the Challenge
To effectively mitigate the impact of adversarial machine learning on malicious software development, organizations must adopt a multi-faceted approach to cybersecurity. This includes investing in robust threat detection and response capabilities, leveraging AI-driven security solutions, and prioritizing employee training and awareness programs.
EXAMPLE:
In 2021 Gartner predicted that by 2025, 30% of organisations will leverage adversarial machine learning techniques to enhance their cybersecurity defences. Furthermore the Gartner Report “Top Trends in Cybersecurity for 2024” predicts that in 2025, 40% of cybersecurity programs will deploy socio-behavioral principles (such as nudge techniques) to influence security culture across the organisation. This constitutes a dramatic rise from less than 5% in 2021. In addition they predict that by 2027, 50% of large enterprise CISOs will have adopted human-centric security design practices.
Our in-house Cybersecurity expert Bernhard Borsch gives the following advice:
“Even small and medium sized enterprises can prepare for this and other new cyberthreats. The judicious use of off-the-shelf and existing software solutions, training of in-house IT experts, and introduction of an Information Security Management System (ISMS) can improve their security posture dramatically. An important mind set to adopt is: Protection is necessary, but a prepared recovery ensures survival!”.
Conclusion
The rise of adversarial machine learning represents a significant challenge for organizations, as it empowers cyber adversaries to develop more sophisticated and resilient forms of malicious software. IT budget holders must recognize the urgency of addressing this threat by not only allocating resources strategically and investing in advanced cybersecurity solutions, but also by employing more human-centric approaches to cybersecurity. By increasing awareness, staying vigilant and being prepared to respond to attacks, organizations can strengthen their defenses and mitigate the risks associated with the accelerated evolution of cyber threats enabled by AML.